- March 10, 2017
- Category: Nuclear Medicine, Scientific Publications
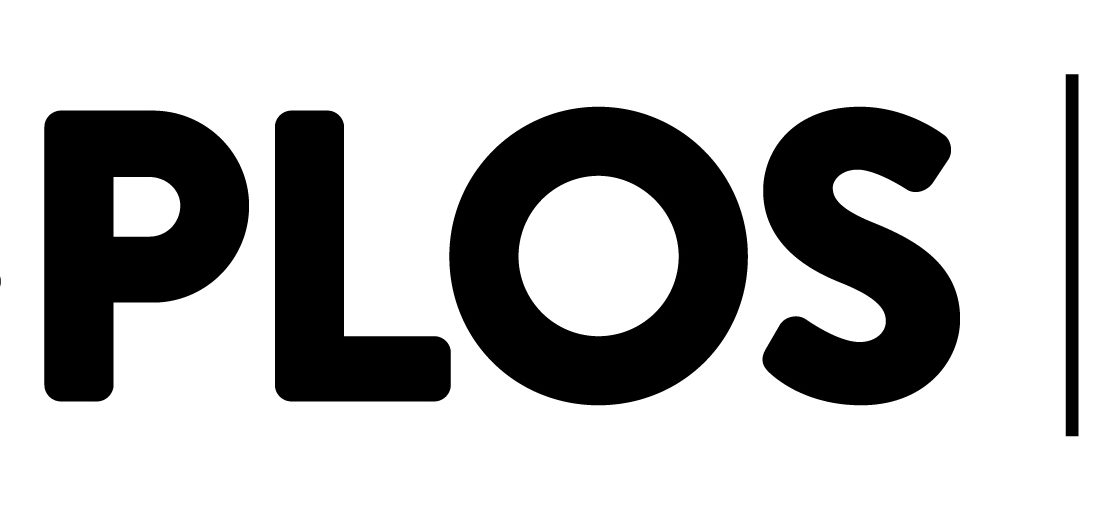
Predictive Value of Initial FDG PET Features for Treatment Response and Survival in Esophageal Cancer Patients Treated with Chemoradiotherapy using a Random Forest Classifier
Paul Desbordes1,2, Su Ruan1, Roman Modzelewski1,3, Pascal Pineau2, Sébastien Vauclin2, Pierre Gouel3, Pierre Michel4, Frédéric Di Fiore5, Pierre Vera1,3, Isabelle Gardin1,3
1 LITIS Quantif-EA4108, University of Rouen, France
2 DOSIsoft SA, Cachan, France
3 Nuclear Medicine Department, Henri Becquerel Centre, Rouen, France
4 Normandie Univ, UNIROUEN, Inserm 1245, Rouen University Hospital Department of Hepato-gastroenterology, Rouen, France
5 Department of Oncology, Henri Becquerel Centre, Rouen, France
ABSTRACT
Purpose: In oncology, texture features extracted from initial positron emission tomography with fluorodeoxyglucose images (FDG-PET) are of increasing interest for predictive and prognostic studies, leading to several tens of features per tumor. To select the best features, we developed a Genetic Algorithm strategy based on Random Forests, called GARF.
Methods: Sixty-five patients with an esophageal cancer treated with chemoradiotherapy were retrospectively included. All patients underwent a pretreatment FDG-PET. The patients were followed 3 years after the end of the treatment. The response assessment was performed 1 month after the end of the therapy. Patients were classified as complete responders and non-complete responders. Sixty-one features were extracted from medical records and PET images. First, Spearman’s analysis was performed to eliminate correlated features. Then, the best predictive and prognostic subsets of features were selected using GARF. The genetic algorithm is based on a fitness function maximizing the accuracy of the RF classification and the value of the area under the receiver operating characteristic curves. The results found with GARF were compared to those obtained by a Mann-Whitney U test (predictive study) and a univariate Kaplan-Meier analysis (prognostic study).
Results: Among the 61 initial features, 28 were not correlated. From these 28 features, the best subset of complementary features found using GARF to predict response was composed of 9 features: gender, usual weight, weight loss, location, tumor stage, metabolically tumor volume (MTV), maximal standard uptake value (SUVmax), skewness, and homogeneity from the cooccurrence matrix. The corresponding predictive value (AUC=0.842±0.099, Se=77±14%, Sp=92±11%) was higher than the best predictive results found using the Mann-Whitney test: busyness from the gray level difference matrix (p<0.0001, AUC=0.810, Se=66%, Sp=88%). The best prognostic subset found using GARF was composed of 8 features: histology, location, WHO stage, nutritional risk index, SUVmax, coefficient of variation, SUV90, and SUV10 (AUC=0.773±0.101, Se=75±17%, Sp=80±16%), while no feature was significantly prognostic according to the Kaplan-Meier analysis.
Conclusion: The GARF algorithm can improve predictive and prognostic values compared to the Mann-Whitney U test and the univariate Kaplan-Meier survival analysis when applied to several tens of features in a limited patient database.
Research Support: This work was partly funded by ANRT (French National Association for Research and Technology) under the contract ANRT n°2013/1153.